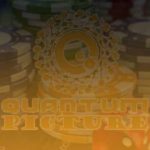
Mengejar hasil taruhan terbesar pada game judi betting liga215 tentunya bisa diwujudkan ketika mulai bergabung dengan agen terbaik. Hampir tiap bandar pilihan pasti membutuhkan banyak hal penting sebagai bukti dalam pelayanan. Semua pemain pasti ingin merasakan berbagai jenis manfaat yang dapat dihadirkan oleh bandar tersebut. Maka, jangan sampai salah untuk menentukan agen pada saat sedang […]